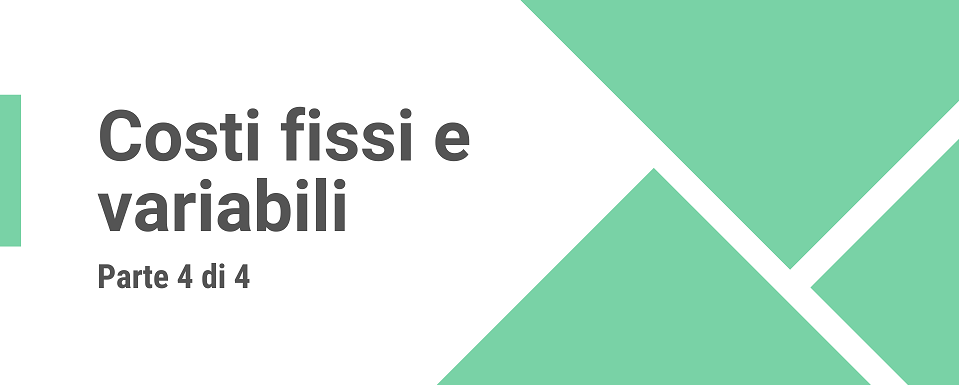
Cost Structure: Ideas for a Practical Calculation - Pt. 4
Share
So far we have seen how to find the function that represents the line of the Total Cost of the department, which expresses on average the dependence of the Total Cost (dependent variable) on the number of pieces produced (independent variable).
Let us now check the goodness of fit of this regression line, that is, let us try to understand if and how much this regression line is useful in representing the observed phenomenon.
To do this, we introduce two simple statistical concepts:
- Regression Deviance, Dev(R)
- Total Deviance, Dev(y)
The Regression Deviance is equal to the sum of the squares of the differences of the theoretical values of the interpolating line with the mean value of the observed dependent variable, or in formulas:
The Total Deviance , on the other hand, is equal to the sum of the squares of the differences of the observed values with their mean value, or in formulas:
These counts were made in columns specifically designed to facilitate calculations, as shown in columns F and G in the figure:
At this point, we can evaluate the goodness of fit of the regression model to the data observed in the 20 weeks through the determination index that we have already mentioned previously, and which is expressed with the indicator R 2 (or R squared ), given by:
Obviously, given how it is constructed, this index will have a value between 0 and 1, and more precisely:
- with R 2 = 0 the statistical model is very bad , that is, the interpolating line is not suitable to represent the observed phenomenon;
- with R2 = 1 the statistical model is excellent , that is, the interpolating line perfectly represents the observed phenomenon.
In our example, the R 2 indicator will be equal to:
which exactly matches the values found previously using both the LINEST formula and the trendline scatterplot.
Once again, after this further review of statistics, it should be noted that, even without setting up all the regression calculations seen so far, to establish whether a certain series of observed data is suitable for interpolation with a sufficient degree of reliability, it is also possible to use other Excel formulas that directly provide the R 2 coefficient considering only the data being surveyed.
These formulas, applied to our example, are RQ and PEARSON, as summarized in the following figure:
Finally, let's try to make sense of this R2 value of 0.8038 that we found in several different ways, and try to grasp its deeper meaning.
Well, the information we can draw from this data is the following:
- the linear relationship of the Total Cost of the department with the number of lamps produced explains 80.38% of the variability of the Total Cost;
- the remaining 19.62% of the variability of the Total Cost of the department is explained by the relationship of the Total Cost with other residual phenomena, other than the number of lamps produced.